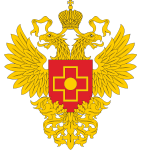
ORIGINAL RESEARCH
Studying visual gnosis through EEG microstate analysis
1 Federal Center of Brain Research and Neurotechnologies of the Federal Medical Biological Agency, Moscow, Russia
2 Institute for Physics and Engineering in Biomedicine, National Research Nuclear University MEPhI, Moscow, Russia
Correspondence should be addressed: Sergey A. Gulyaev
Ostrovitianova, 1, str, 10, Moscow, Russia; moc.liamg@37veaylug.s
Compliance with ethical standards: the study was approved by the Ethics Committee of the Federal Center for Brain and Neurotechnologies of FMBA (protocol № 148-1 dated June 15, 2021) and carried out in accordance with the principles of biomedical ethics set out in the Declaration of Helsinki (version issued in 1964 and the next updated versions). The informed consent was submitted by all study participants.
Establishing a link between the objective research data and the thought process is the most important issue of modern neurophysiology.
Following the development of the method for human electroencephalography recording by H. Berger in 1929, the researchers proposed different approaches to the issue, from attempting to convert brainwaves into audio recording [1] to the use of top-down artificial intelligence system that involved using the data sets obtained when examining people with various disorders. However, despite the range of the used technologies, we are still a long way from resolving the problem.
Currently, researchers are less interested in neurophysiological techniques based on EEG/MEG recording of various modalities due to a number of objective reasons. Thus, conventional EEG tests involve recording of continious rhythmic activity of the brain with the pool of electrodes evenly distributed over the scalp surface. All electrodes are equal in design and function, that is why a composite signal resulting from the activity of brain structures together with multiple artifacts of physiological and physical origin are registered on each electrode [2]. There is a need for careful processing of the composite signal to distinguish distinct components by mathematical methods [3] that require in-depth training of specialists. The other feature of the technique is that it is difficult to establish an objective link between the characteristics of the studied signals and the changes in environmental conditions, i.e. signal sensitivity. Discrete methods, such as evoked potential tests, involve recording the changes in biopotentials after stimulus presentation, thus allowing the researcher to set the zero reference point to assess the association of the changes observed with significant and non-significant stimuli. However, a wide range of different stimuli is needed to assess a complex cognitive stimulus, that is why different method implementation is required [4, 5].
Among EEG data processing methods designed for this purpose that make it possible to establish an objective link between the changing characteristics of the signal recorded and external exposure, clustering algorithms [6] and techniques for solving the inverse EEG problem [7–9] are the most interesting. These methods allow for determining the pattern and detection of changes in bioelectric activity of distinct brain structures.
Currently, clustering of continuous EEG signal proposed in the 1990s [10] enables real-time acquisition of data from the neuronal structures of the brain. The technique is based on monitoring the characteristics of distribution of the head surface biopotentials that show discrete changes in the total energy density of the total scalp potential. Thus, the first variant remains stable within a short time frame, then it quickly gives way to the other one that also remains stable for some time. In 1995 R. Pasqual-Marque introduced a mathematical method for active detection of EEG microstates by clustering continuous EEG data [11, 12]. These studies confirmed the perception that each EEG microstate reflected the function of certain neural network of the brain or functionally linked group, and microstate sequences could be considered a reflection of realization of certain brain function [13]. Such approach has made it possible to divide the continuous flow of EEG data acquired during initial assessment into components that currently allow us to detect as many as 39 distinct EEG microstates. However, only six first microstate classes can be fully representative, which is possibly due to the activity of large neural networks responsible for realization of basic and the most stable brain functions; impairment of these functions manifests in the form of severe mental disorders [14–16]. Therefore, the functional study performed using the method outlined above makes it possible to define interconnection system, and the technique for solving the inverse EEG problem allows one to map brain structures involved in implementation of the studied brain function. However, when using the technique for solving the inverse EEG problem, it is necessary to take into account that the terms “EEG activity” and “functional activity of nervous tissue” are not synonymous in the context of physiological research [17]. When performing physiological research, the power of scalp potential of the cortical areas that produce rhythmic activity is registered as the area of high signal strength in contrast to the areas of the nervous tissue excitation characterized by low amplitude and disorganized EEG patterns [18, 19].
That is why the use of combined analysis based on various functional imaging techniques (EEG-fMRI, MEG-fMRI, etc.) to confirm the physiological research data could be ineffective [20]. The study was aimed to develop a system for analysis and assessment of visual gnostic processes as a model of higher nervous function by solving the inverse EEG problem based on the cluster EEG microstate model.
METHODS
A total of 30 generally healthy people aged 30–60 (32.4 ± 9 years) were enrolled. Inclusion criteria: no significant visual problems. Exclusion criteria: history of head or eye injury; taking medications on a regular basis. The resting state EEG was continiously recorded in all subjects with their eyes closed, eyes open, and when continiously looking at symbols (letters, numbers) in the black and white contrast image on the computer screen. The subjects were allowed to rest for 3 minutes between tests to recuperate.
Subsequent analysis of encephalograms included removal of artifacts resulting from interference during EEG recording by filtering using digital filters with fixed bandwidth of 1–35 Hz in order to suppress the galvanic skin response and myographic artifacts. Later physiological artifacts were removed using the independent component analysis performed with the RUNICA utility of the EEGLAB Ver. 2022a software package (USA). Clustering of the continious EEG signal was performed by distinguishing distinct stable EEG microstates in accordance with the selected six-component model using the atomize and agglomerate hierarchical clustering (AAHC) that showed higher sensitivity compared to K-means clustering. Six components were selected based on the current two-streams hypothesis of functional brain network organization and the existence of functional links between certain microstates and information processing. In this case two additional microstates were considered the reserve quantities. Spatial localization of rhythmic activity for each EEG microstate was calculated at the final stage using the algorithms for solving the inverse EEG problem implemented in the sLORETA software package.
Histograms of bioelectric activity recorded within Brodmann areas were created based on the results of each test for each participant and each group (average).
Hardware and software used during the study
A 128 channel EGI-GES-300 bioamplifier combined with the original GSN-128 sensor net system (analogue for the 10-5 system) (MAGSTIM; USA). EEGLAB software package for MATLAB_ Runtime 2021b (Center of the Institute for Neural Computation, the University of California San Diego; USA). eLORETA v20210701 software package (University Hospital of Psychiatry, University of Zurich; Switzerland). Statistical software package GNU PSPP (International). Operating system Linux Mate 21.10 (Canonics; UK). Suite of office applications LibreOffice 7.2 (Document Foundation; USA). No commercial licenced software was used during the study.
Selection of functional study design
The study involved stimulation of the visual sensory system since its neuronal networks occupied the largest area of the cortex, and excitation in these networks caused the most significant changes in rhythmic activity of the brain thus allowing one to get more accurate results compared to analysis of activity of other neuronal systems. The following situations were chosen as loading tests: the state of relaxed wakefulness with eyes closed or open, and the state of visual gnosis realization through looking at various symbols and signs on the screen of LED monitor. Our aim was to define the characteristics of bioelectric activity with the sensory system in inactive (eyes closed) or active state, in the situation of no mental task performed (relaxed wakefulness with eyes open) or during realization of mental task in the form of test that involved looking at symbols.
Statistical analysis
A linear model that included one factor, the impact on the visual sensory system, was used in the study. Regarless of the direct correlations between the experimental data, one-way analysis of variance (ANOVA) was chosen after testing for normality due to the differences between data obtained using the model for dividing the studied activity into classes. Significance of the results obtained by solving the inverse EEG problem was tested using the Pearson's chi-squared test, since the changes were of a qualitative nature. Assessment was performed in accordance with the guidelines [21].
RESULTS
Characteristics of EEG microstates obtained during the experiment
The main objective measures of EEG microstates used in the study were as follows: microstate occurrence per second, microstate duration in seconds, and microstate percentage in the structure of the total EEG potential (coverage). These indicators conditionally reflected the characteristics of certain neuronal network that had generated every single microstate, the frequency of its functional activation during realization of the studied function, and relative amount of functional elements contained in this network.
Studying the EEG microstate occurrence showed that the biggest changes took place when comparing the tests performed in the state of relaxed wakefulness with eyes open with the tests performed with eyes open. Thus, indicators of the first and second class significantly (p < 0.05; ANOVA) changed during all tests. However, in other classes of EEG microstates, significant differences (p < 0.05; ANOVA) were observed only with the state of eyes-closed relaxed wakefulness. In other cases no significant differences in occurrence were found when peforming tests with eyes open (0.1 < p < 0.8; ANOVA) (fig. 1).
Calculation of the EEG miscrostate contribution to the total energy of the scalp field (coverage) revealed no signficant differences between the characteristics obtained during three tests. The significance level exceeded 0.5 (fig. 2).
Assessment of EEG microstate duration (fig. 3) showed that this indicator significantly increased when the subjects opened their eyes or performed the loading test that involved looking at symbols (0.01< p < 0.05; ANOVA). Comparison of the values obtained when the subjects had their eyes closed or were actively engaged in performing the visual test revealed almost no differences (0.1< p < 0.9; ANOVA).
Solving the inverse EEG problem for the used model of the EEG microstate classes
The inverse EEG problem was solved using the e-LORETA software package. Source localization and verification against Brodmann's map was performed by clustering for each EEG microstate class.
In the state of relaxed wakefulness with eyes closed, activity was detected within the following Brodmann areas: 11 — areas of olfactory system, 18 and 19 — secondary zones of visual sensory system, 21 — vestibular area, 37–47 — areas responsible for perception of music. In the context of detecting rhythmic EEG activity these reflected the centers' readiness to perceive and analyze the tonal stimuli, or to respond in case of human reaction to stimulation in the form of sound (screaming, sounds having no morpho-phonetic structure).
Eye-opening changed the pattern recorded. Three main zones of activity were distinguished: area 18 — areas of visual sensory system responsible for the written text recognition, area 20 — cortical center of the vestibular system / complex pattern recognition, area 37 — acoustic-gnostic speech center.
When performing the test for visual gnosis that involved looking at written symbols, activity was detected within a larger number of Brodmann areas compared to the state of relaxed wakefulness (fig. 4). Activity was found in Brodmann areas 18 and 19 responsible for visual perception of images, area 39 being a part of Wernicke's area, and the structures of premotor and prefrontal areas (areas 6–11).
DISCUSSION
The study showed that the technique for continuous EEG monitoring supplemented by methods for EEG microstate analysis and solving the inverse EEG problem could be used as a research tool for assessment of changes in functional brain activity during realization of higher nervous functions.
Analysis of the main EEG microstate characteristics has shown that activation of visual sensory system has an overall impact on the occcurence and duration of each EEG microstate class. However, no significant changes in the contribution of each class to the total energy of the scalp field (coverage) are observed. This fact correlates with the results of a number of studies [22, 23], since such stability results from intact structure of neuronal networks involved in generation of each microstate in the generally healthy experimental subjects.
Significant variability of the first and second class EEG microstate occurrence was also the expected result that had been previously described in literature. Such variability was related to rearrangement of large neuronal network during realization of functional response. As previously reported, the lack of similar dynamic changes in the characteristics of other four classes was possibly due to the fact that these were more difficult to distinguish when assessing the recording [24].
Observations suggest that microstates detected when the subject is in a state of relaxed wakefulness with his/her eyes closed or in a situation of visual load are not identical, since the activity of neuronal networks that generate these microstates shows up significant differences in both occurrence and duration of each of the distinguished EEG microstate classes. It may be assumed that the posiibility of EEG signal discretization involving division into separate groups of brain activity that correspond to functioning of distinct neuronal networks is the main effect of the used cluster model. However, calculation of basic EEG microstate characteristics makes it impossible to define their specificity.
The experiment showed that EEG microstates detected when the subject was in a state of relaxed wakefulness or in the situation of visual load were not identical. Rather these were gauge derivatives of clustering in the context of used mathematical model, which was confirmed by the differences in their basic characteristics measured in a resting state, with eyes open, and when performing the loading test. However, no reports of similar findings were found in the reviewed literary sources.
Solving the inverse EEG problem at the final stage of the study made it possible to localize and define (based on Brodmann areas) three average sequences typical for the loads used during the study. Taking into account the features of the EEG signal recording and frequency analysis, these reflected the processes of preparing/selecting the appropriate neuronal networks for further functional activity. Such processes can be considered the idle state [25–27]. However, during realization of complex (gnostic) brain functions, excitation and idling processes change each other in a rhythmic manner, thus allowing us to characterize certain sections as active and consider these as related to realization of the studied function. Thus, in the state of relaxed wakefulness with eyes open almost no rhythmic activity was detected within the Brodmann area 19, since this neuronal network was in the excited state and showed minimum bandpower of the signal. At the same time bandpower of the areas responsible for complex pattern recognition (area 20) and gnostic centers of sound perception and analysis (area 37) indicated that these structures were ready for engagement, which actually corresponded to the state of increased attention while awaiting speech addressed to a person. No such components related to being more focused on a specific task were observed in a state of passive wakefullness with eyes closed. The changes in rhythmic activity affected visual areas (areas 18 and 19) and the center involved in processing of music (area 47), which corresponded more closely to awaiting perception of external signals, readiness for analysis of these signals and primary non-specific response. Under the test load in the form of looking at symbols the patterns of rhythmic activity were more complex than in the state of passive wakefulness, since these involved a larger number of Brodmann areas. This observation was considered as a consequence of the neural network cyclic transitions between the excited state and idle state during realization of visual gnostic function.
Thus, we believe that the method proposed in our study based on using EEG microstates makes it possible to reveal functional sequences associated with realization of distinct brain functions. In the future the method may be used in developing the new diagnostic equipment for objective assessment of thought processes in the brain.
CONCLUSIONS
The study has shown the potential of studying higher nervous functions by recording the changes in characteristics of the scalp surface biopotentials. However, implementation of the technique requires a radical revision of both EEG test organization and analysis of the results. It is necessary to develop the new diagnostic equipment that should be quite different from the currently used conventional EEG systems. The analytical unit should be completely revised in favor of systems for automated analysis of activity generated by structures in the brain. The prospects of such inventions are not only of great interest for modern neuroscience, but also have a significant potential for the development of human monitoring systems in harsh environmental conditions.