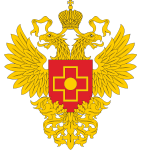
METHOD
Method for quantitative assessment of protective immunity against SARS-CoV-2, its duration and antibody dynamics
1 Centre for Strategic Planning and Management of Biomedical Health Risks of the Federal Medical Biological Agency, Moscow, Russia
2 Military Academy of Radiation, Chemical and Biological Defense, Kostroma, Russa
Correspondence should be addressed: Alexandr M. Karmishin
Shchukinskaya, 5/6, Moscow, 123182; ur.zmpsc@nihsimraka
Author contribution: All authors equally contributed to the methodology of the study, data acquisition, analysis and interpretation. All author participated in drafting the manuscript and editing its final version.
In December 2019, an outbreak of the respiratory disease caused by a novel coronavirus was reported in China. The infection rapidly spread across the globe. On February 11, the disease received its official name COVID-19 from WHO; at the same time, the International Committee on Taxonomy of Viruses named the novel pathogen SARS-CoV-2 [1]. On March 11, WHO declared a pandemic of the novel coronavirus disease.
SARS-CoV-2 is a representative of the Betacoronavirus genus from the Coronaviridae family. The virus has 4 major structural proteins: a spike protein (S) consisting of 2 subunits S1 and S2; an envelope protein (E); a membrane protein (M), and a nucleocapsid protein (N). The receptor-binding domain (RBD) of S1 mediates SARS-CoV-2 entry into the host cell. The nucleocapsid protein plays a significant role in viral transcription and assembly in the host cell. As a rule, neutralizing antibodies bind to the RBD of the S protein blocking the entry of the virus into the cell. At the same time, the mechanisms of interaction between the antibody and the N protein are yet poorly studied [2, 3].
During the first phase of modeling the COVID-19 epidemic, a method was proposed for determining the quantitative characteristics of SARS-CoV-2 spread in different countries [4–7].
According to experimental data, the dynamics of antibody production against any pathogen, including SARS-CoV-2, after exposure or immunization are characterized by the following pattern [2, 3, 8]:
- the antibody titer rises;
- in a general case, the titer reaches a plateau level;
- the titer gradually wanes, falling to 0 in a general case or to some background value in a special case.
Immunity is a state in which the organism is not susceptible to infection. Almost every immunity can be overcome with massive doses of pathogen. Protective immunity can be measured as “the protective titer of neutralizing antibodies”, i.e. the level of specific antibodies in the blood serum ensuring protection against the disease, which may develop following exposure to the pathogen. The “protective titer” is a relative concept. Notably, low antibody titers may confer some protection against the pathogen, whereas high antibody titers do not guarantee absolute protection against the disease [9].
This definition of protective immunity is very general and does not account for its quantitative characteristics.
Since the development of an infectious human disease is a stochastic process, protective immunity can be defined in stricter terms. It is the level (titer) of antibodies at which the probability of disease after exposure to the infective dose does not exceed a specified (forced) probability.
The duration of immunity is the time span during which protective immunity is sustained.
In order to calculate the protective level of antibodies and the duration of protective immunity, a mathematical model is needed that would describe the probability of an infectious human disease at a given antibody titer and antibody persistence after exposure to the infective dose. So, the problem of describing protective immunity in quantitative terms can be broken down into two interrelated problems:
- to mathematically reason the relationship between the dynamics of parameters of the hazard factor law, which describes the probability of infection in the human population, and the levels (titers) of antibodies against this infection;
- to build a mathematical model of antibody titer dynamics in a human organism.
So far, the level and duration of immune protection against SARS-CoV-2 following infection or immunization remain unknown; this fact is openly admitted by the developers of Russian and foreign vaccines and specified in the instructions for their use [10–14].
The aim of this study was to develop a mathematical model for quantitative determination of protective immunity against SARS-CoV-2 and its duration.
METHODS
The following theoretically or experimentally established factors were considered while developing a mathematical model of antibody titer dynamics:
- all else being equal, the probability of an infectious human disease depends on the antibody titer: the higher the titer, the lower the probability;
- antibody production is a stochastic process; consequently, the levels of a given antibody type will differ among individuals at a specific point in time following exposure to a given infective dose; in other words, antibody titers are a random variable;
- in a human organism, antibody titers rise, then reach a plateau level and then wane to 0 or some background value;
- protective immunity and its duration are determined by the level of specific antibodies.
Based on the methodological and scientific consensus, this study attempted to build a mathematical model describing the stochastic character of the novel coronavirus disease following exposure to its causative agent and the dynamics of antibody titers to SARS-CoV-2. This model could be instrumental in solving a number of important practical tasks, such as determining the level of protection against the infection and the duration of protective immunity.
This study used literature data on the results of testing for IgM and IgG against the S and N proteins and the RBD-fragment of the S-protein conducted on 1,850 patients hospitalized for COVID-19 [2]. Figures were prepared in Microsoft Office Excel 2013 (Microsoft; USA).
RESULTS
Using methods of differential and integral calculus and the probability theory, we developed a mathematical model describing the dynamics of antibody titers in human blood in order to solve the following tasks:
- to predict the dynamics of antibody titers in an infected or immunized population;
- to estimate the duration of an infectious disease that is at or above a specified severity level;
- to estimate the level of protection and the duration of protective immunity;
- to provide a rationale for vaccine requirements considering the purpose of vaccination.
It follows from fact 1 (the probability of an infectious disease depends on the antibody titer) that at any level of specific antibodies, e.g. IgG, the disease is probable; therefore, a bivariate probability density function for a random antibody titer and an infective dose causing the disease at this titer can be added to the analysis. Based on the previous theoretical and experimental research [4, 6, 7, 15], we conclude that the probability density function is described by a bivariate lognormal distribution:
where ID50 is a median of the random infective dose that causes a studied infectious disease at a titer Т50 with 0.5 probability; Т50 is an antibody titer median observed in biological objects of the same type (homogenous groups of humans); σlnD, σlntT a are mean squared errors of the natural logarithm of the random disease-inducing infective dose and the antibody titer, respectively; rlnDlnT is a correlation coefficient for the natural logarithms of the random variables in question.
In the general case, the domain of the correlation coefficient is [–1; 1], but in our case the correlation coefficient must be positive and defined in the interval (0; 1] because the diseasecausing infective dose is expected to increase as the antibody titer rises.
It follows from equation (1) that if titer T of IgM or IgG antibodies is a fixed value, then the random infective dose that causes a mild, moderate, severe or very severe infection will have a log-normal distribution:
where ID50* is a distribution law parameter: the median value of the random infective dose that causes a mild, moderate, severe or very severe infection at a given antibody titer; σIn*D is a mean squared error of the natural logarithm of the random infective dose that causes infection at a given antibody titer.
Parameters of the conditional probability distribution of a random variable D͂ from the equation (2) can be expressed using the parameters of bivariate density distribution (1) and the fixed value of the antibody titer as follows [16, 17]:
From the conditional probability distribution of the random infective dose that causes the infectious disease of or above a specified severity level at a given antibody titer follows the socalled hazard factor law [4, 7], which describes the probability of a studied infectious disease at antibody titer T depending on the applied infective dose:
ID50* is a parameter of the law: the infective dose at which the probability of the onset of an infectious disease of severity at or above the specified level (mild, moderate, severe, very severe) at a given antibody titer equals 0.5.
The relationship between the hazard factor law describing an infectious disease of severity at or above the specified level and the titer of IgG antibodies is schematically shown in fig. 1.
It follows from the hazard factor law that the infective dose at which the infectious disease develops with the probability p can be defined as shown below [15]:
where argerf(u) is a function inverse to the integral of error function [13].
Thus, in order to determine the probability р of developing an infectious disease and the probability 1–р of not developing this infectious disease after exposure to the infective dose D, we need to know the point in time after exposure when the IgG antibody titer will equal T.
When talking about the probability of developing or not developing an infectious disease, a safe infective dose can be introduced to the analysis. By analogy with the safe dose of toxic chemicals [16, 17], the safe infective dose of a pathogen can be defined as follows: it is an infective dose at which, at a given antibody titer, the probability of developing the disease does not exceed a given probability (forced or psychologically acceptable).
A study [4] demonstrates that IgM and IgG titers measured in different individuals at the same point in time after exposure vary.
According to the existing theoretical concepts, the level of any antibody type at a specific point in time after exposure is a continuous random variable defined in the interval [0, ∞]) and characterized by a log-normal unconditional distribution inferred from equation (1). Then, the probability of a random variable (antibody titer) at a certain point in time after exposure being less than Т can be described as follows:
where Те is a distribution parameter for a random antibody titer: its median (half of the people at a given point in time after exposure will have antibody titers below Те, the rest will have antibody titers above Те); form. 21 is a distribution parameter characterizing the range of antibody titers relative to the median (the lower form. 21 , the wider the range relative to the median and, conversely, the higher form. 21 , the narrower the range).
Distribution parameters for a random titer (6) can be defined from experimental data using a previously proposed method In some coordinate system, equation (6) represents a line [7]:
therefore, the method of least squares can be employed to estimate the unknown parameters of equation (7).
Table 1 (tab. 1) shows grouped data on IgМ titers on day 7 after exposure and IgG titers on day 33 after exposure (raw date were taken from [2]).
The results of the analysis based on the method of least squares of data from tab. 1 for equation (7) are provided in tab. 2; fig. 2 shows the linear representation of these data.
Parameters of distribution for random antibody titers at other points in time after exposure were estimated in a similar fashion.
Satisfactory estimates obtained during the analysis support a theoretical conclusion that a random titer at a given point in time after exposure has a log-normal distribution.
Table 3 (tab. 3) shows the results of the statistical analysis of IgM and IgG titers described by equation (7) which was conducted based on the method of least squares: distribution parameters for random Te and form. 21 and statistical estimates of equation parameters and the equation itself: Fisher’s criterion, the correlation coefficient and mean squared errors for lnTe and form. 22 .
The analysis of the dynamics of median antibody titers shows that the medians increase at first, reach a plateau level and then wane, falling almost to zero. Pharmacodynamically, this pattern can be easily explained within the confines of a one-compartment model [15]: after exposure, the organism starts to produce antibodies at a fixed rate; at the same time, the level of the produced antibodies decreases due to antibody elimination mechanisms, including metabolism, excretion of antibodies in the unchanged state, etc. At some point, when the rate of antibody production reaches the rate of their elimination, the dynamics of antibody titers will reach a plateau. Once the secretion of antibodies stops, their concentration in the blood will fall exponentially. Such qualitative dynamics of antibody titers allow us to arrive at the following differential equations.
In a time interval from 0 to the cessation of antibody production (τ), a change in the antibody titer dT during an infinitely small time interval can be described as:
The solution to this equation takes the form of:
where q is the rate of antibody production; Vk is the total blood volume in the organism, ml; λ is the immunoglobulin elimination constant expressed as day–1.
Since q equals 0 once the secretion of antibodies has stopped (t > τ), differential equation (8) will take the following form:
equation (10) can be written as follows:
Parameters of the model (8–11) were determined using the numeric method of least squares in Excel.
Fig. 3 (fig. 3) shows the dynamics of median IgM titers and a theoretical curve described by equations (9) and (11).
The proposed mathematical model is useful for solving a range of applied problems, like computing the level and duration of immune protection following exposure to the infective dose.
Let us formulate the problem. There is a need to determine the level and duration of protective immunity after exposure to the infective dose D, given that the probability p of developing the infectious disease does not exceed a given (forced) probability.
Based on the hazard factor law (4), by analogy with equation (5), let us find ID50 at which the probability of the disease after exposure to the infective dose D equals р:
Having found ID50* (T) let us now find, based on the bivariate log-normal distribution, the IgG titer at which ID*50 (T) is:
Earlier in this paper, we showed that at a given point in time after exposure the IgG titer is a continuous stochastic variable which follows a log-normal distribution. So, there is some probability that this titer will be equal to or be higher than T (the protective titer). Assuming there is a sufficiently high probability p (T) that the antibody titer is equal to or higher than T, let us find a median titer which ensures this probability:
Then the probability of an individual not developing the disease after exposure to the infective dose D is the product of multiplication of 2 probabilities: the probability р (T) that the IgG titer will be higher than T and the probability 1–р (D) that the infected person will not develop the disease after exposure to the infective dose, i.e.:
It follows from this solution that for humans with a given antibody titer, the level of protection (protective immunity) is linked to the probability of developing or not developing a disease disease following exposure to some infective dose D.
Now, let us find the duration of protective immunity, i.e. the time interval thoughout which the IgG titer equals to or is above a given value. This is consistent with the median Те titer being equal to or above the value determined by equation (14).
By equaling the median titer Те, determined by (14) to its dynamics over time, we will get:
- at t < τ
- at t > τ
The onset time to and the termination time tt are inferred from equations (16) and (17). Those are time points after exposure at the beginning and at the end of the time interval throughout which the median titer is maintained at the level equaling to or higher than a given level determined by expressions (16) and (17):
The graphic representation of these time values is provided in fig. 4.
Considering the abovesaid, the duration of protective immunity can be calculated using the formula:
These solutions work for different types of antibodies produced after exposure to a pathogen or after immunization.
In the most general case, parameters of the proposed models depend on a number of factors like sex, age (children, adults, individuals of advanced age), general health (seemingly healthy individuals, patients with chronic conditions, etc.), medical history (a past history of the disease or immunization, immunized patient with or without a past history of infection).
In order to quantitatively determine the level and duration of protective immunity, we need to know 5 quantitative characteristics of virulence for each of homogenous population groups in a system of 2 random variables: the infective dose and the antibody titer at which the disease develops, as well as to describe the dynamics of antibody titers after infection or immunization.
DISCUSSION
As a rule, the literature provides only qualitative or semiquantitative estimates. Often, there is no characterization of antibody titers at some point in time after exposure: positivity coefficients are described instead. Usually, no theoretical rationale for antibody titer dynamics is provided [2, 3, 8, 18, 19].
The dynamics of antibody titers are different for different antibody types. For example, the rates of production of IgM and IgG antibodies to the N-protein and RBD are not the same. IgM antibodies to the N protein are produced at a faster rate and have a high peak, whereas IgG-antibodies for the same protein are produced at a slower rate and do not reach high concentrations. By contrast, IgG antibodies to RBD grow rapidly, whereas IgM antibodies to RBD are produced at a slower rate [18, 19]. Most of the existing ELISA kits for measuring antibodies to SARS-CoV-2 target the S-protein, its RBD fragment and the N protein.
CONCLUSIONS
A few mathematical models were developed in the course of this study: 1) the hazard factor law, which models the probability of developing COVID-19 after exposure to the infective dose of SaRS-CoV-2 by an individual who already has some IgG; 2) the model of antibody titer dynamics in a human organism based on the averaged rate of antibody production over time — and the rate of simultaneous antibody elimination by all bodily mechanisms; 3) using these 2 models, a method for measuring protective immunity and its duration was proposed. Similar solutions can be applied to immunized populations. In order to obtain hard numbers reflecting the level and duration of protective immunity, parameters of the proposed models need to be experimentally identified first. This will help to bridge the existing gap associated with the absence of one of the basic vaccine characteristics: developers of SARS-CoV-2 vaccines do not know what antibody levels and immunity duration are protective against the disease.