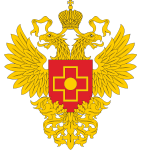
ORIGINAL RESEARCH
Probability of infectious disease in humans during epidemic
1 Centre for Strategic Planning and Management of Biomedical Health Risks of the Federal Medical Biological Agency, Moscow, Russia
2 Federal Medical and Biological Agency, Moscow, Russia
Correspondence should be addressed: Alexandr M. Karmishin
Shchukinskaya, 5/6, Moscow, 123182; ur.zmpsc@nihsimraka
Author contribution: All authors equally contributed to the methodology of the study, data acquisition, analysis and interpretation. All author participated in drafting the manuscript and editing its final version.
The COVID-19 pandemic, which began in China in late November/early December 2019 [1], has raised the need for an adequate mathematical model that would accurately forecast epidemiological metrics, including the total number of cases and deaths, timeline, etc. Predictions generated by popular SIR models [2, 3] and their modifications turned out to be wrong because such models are based on false assumptions about how infection develops both in an individual and in the entire population. For example, such models predict the number of infected individuals at a given point in time from the number of contacts made by susceptibles and infectives, but not from the infective dose, which actually determines the probability of infection.
Similar to industrial accidents at hazardous facilities [4, 5], an epidemic should be described at 3 interrelated levels of generalization:
- the low generalization level (with a focus on a host-pathogen interaction), which involves a) providing mathematical reasoning for the laws describing how a pathogen or a group of different pathogens establish an infection in a human or a nonhuman biological object and b) virulence assessment for each route of entry into the host;
- the medium generalization level (with a focus on the transmission/spread of a studied infection in a population), which describes the infective dose received by a human or a non-human biological object via each route of transmission;
- the high generalization level, which describes the integral spatiotemporal parameters of infection spread.
This article focuses on the first (low) generalization level, i.e. infection with one pathogen type.
The aim of this study was to find the laws describing the probability of infection in a human or a non-human biological object.
METHODS
The laws describing how infection is established in a human or a non-human biological object can be constructed theoretically or from experimental data. This article presents the results of theoretical research.
Importantly, the main quantitative characteristic of a pathogen that determines the probability of infection or death of a biological object is the infective dose D, as opposed to contact between a susceptible and infective individuals.
Similar to the concept of the toxic dose of toxic chemicals [6], an infective dose is the amount of pathogen (a biohazardous agent, BHA) entering the organism. This dose can be expressed in BHA mass units or special units like CFU (colony forming units), PFU (plaque forming units) and au (arbitrary units).
In order to find the laws describing how infection is established in a human or a non-human biological object, the following situations should be considered:
- exposure to different infective doses of one or a variety of pathogens, temporal characteristics of the infection not being accounted for;
- exposure to different infective doses of one or a variety of pathogens, time to onset of signs and symptoms being factored in;
- exposure to different infective doses of one or a variety of pathogens, time to onset t and duration τ (time to recovery) being factored in.
Obviously, the second situation is more general than the first, and the third situation is more general than the first two.
Graphs included in the article were constructed in Microsoft Office Excel 2013 (Microsoft; USA).
RESULTS
Using the toxicity of chemical agents or pharmaceutical drugs as an analogy [6], the simplest problem, in our case, can be set up as follows.
Let us assume that when a pathogen gains access to a given host type (e.g. an adult human) via a given route of entry, it is expected to produce a specific effect (a mild, moderate, severe or critical infectious disease). Because humans differ in their immune status, the infective dose needed to produce this effect will vary between the exposed hosts. Therefore, the amount of pathogen capable of producing a certain effect (evoking a certain response) can be considered a continuous random variable.
According to the probability theory, a random variable is best described by its distribution law; the distribution law of a continuous random variable can be described by:
- a uni- or multivariate probability density function;
- a probability distribution function of the considered random variable (integral function).
The probability density function φ(D͂) of the random infective dose (ID) value D which elicits a certain response in a human or a non-human biological object is shown in fig. 1.
By definition,
where dN is the number of objects for which the random ID value eliciting a certain response falls within a range between D͂ and D͂ + dD͂;
N is the total number of objects;
dN/N is the proportion of objects for which the random ID value evoking a certain response ranges from D͂ to D͂ + dD͂; if N is relatively high, this proportion can be interpreted as the probability dP of the ID that evokes a certain response falling within the range between D͂ and D͂ + dD͂; so
By definition, the distribution function (fig. 2) describes the probability of the random ID value that elicits a certain response in a biological object being lower than D, i.e. F(D) = P (D͂ < D).
Then, if a biological object is exposed to some infective dose D at Р = F(D), the random ID value capable of causing infection in this biological object will be lower than the applied dose; so, the harmful effects of the pathogen will not be below a given level at Р = F(D) (fig. 3).
Similar to toxic chemicals leaking during industrial accidents [6–8], the following definition can be given:
The relationship between the probability of infection whose severity is not below a given level and the infective dose is called the hazard factor law (HFL).
In general, the integral representation of this law takes the form of:
This law is schematically shown in fig. 3. Its specific representations are based on the data generated by experiments on animals. If an object is exposed to multiple hazards, the subintegral function from expression (1) can be represented by the normal or log-normal distribution, the Weibull or gamma distribution, or approximations by linear equations, the logistic curve, etc. [5].
It should be noted that when experimental data are processed, it is often impossible to favor one type of distribution because the obtained experimental data conform to different types of distribution.
However, when more than one random variable is included in the equation (the infective dose, time to onset of symptoms, duration of the disease), i. e. we deal with a multivariate random variable, the situation with the distribution type clears up.
Let us consider exposure to one or a variety of pathogens and account for time to onset of mild, moderate, severe or critical symptoms.
According to experimental data, time between exposure to a given infective dose and onset of clinical symptoms of various severity (the incubation period), including death, is a random variable (conditional probability distribution of one random variable in the presence of another fixed variable). Typical time to onset characterized by mathematical expectations, modal or median times correlates with the actual exposure dose.
By analogy with some other studies [6–8], we conclude that the infective dose evoking a specific response and time to its onset are continuous correlated random variables. The probability density function φ(D͂,t͂) for such two-dimensional random variables is shown in fig. 4. In practice, it is often required to calculate the probability of infection developing by a certain point in time t or the likelihood of death. This metric can be calculated using the formula [5–8]:
Expression (2) is a common integral representation of a solution to the problem of determining the probability of developing symptoms at or above the specified severity level by some point in time t depending on the infective dose; it is referred to as the hazard time-factor law (HTFL).
Thus, this law describes the probability of developing an infectious disease at or above the specified severity level by the point in time t depending on the actual infective dose (fig. 5) [8].
In a special case, if time of onset from expression (2) approaches infinity, the expression takes the form of the hazard factor law (1). Therefore, at this stage of analysis we arrive at the conclusion about the form of subintegral functions from expressions (1) and (2).
The only known type of distribution for continuous random variables existing under the probability theory is the normal type. However, it cannot be used to solve the problem set in this paper because the normal distribution domain (–∞; ∞) does not coincide with the domain of random variables [0; ∞]. There are no laws of multivariate Weibull distributions, gamma distributions or the like that could, in a limiting or special case, produce a Weibull or gamma distribution or a Weibull-gamma distribution [5].
About 15 years ago, we were working on a mathematical model describing the combined effect of bioactive substances, such as pharmaceutical drugs and toxic chemicals, and discovered a multivariate log-normal distribution of continuous correlated random variables [6]. Now, in the case of death from infection, the bivariate probability density function from expression (2) can take the following form:
If an object is exposed to a fixed amount of pathogen (infective dose), then time to onset of symptoms at or above the specified severity level (incubation time) is a continuous random variable characterized by log-normal distribution [5–8]. The conditional probability distribution of random time to onset (for symptoms at or above the specified severity level) describing the probability P(t) of this random time being shorter than time t will take the following integral form:
These parameters are defined using quantitative characteristics of pathogen virulence and the actual infective dose as shown below [6, 8]:
According to experimental data, the amount of pathogen that causes a disease in a human biological object, disease incubation time and duration (time from onset of clinical symptoms to recovery) are continuous random variables.
In 2007, it was demonstrated that the probability of the harmful effect (which, in our case, is infection) that is not less than a given severity by the time t and for a duration τ (that not less than a given one) can be defined as follows [8]:
where f(D͂,t͂,τ͂) is a 3-variate log-normal distribution density of continuous correlated random values: the infective dose D͂ capable of causing infection at or above the specified severity level, time of onset t͂ and duration of infection τ͂, characterized by 9 parameters, which, in the case of a pathogen, are quantitative characteristics of pathogen virulence
This probability is referred to as generalized HTFL [8].
If duration of an infectious disease is not included in the equation, as is the case with deaths from infection, then, assuming that (6) τ = 0, we will arrive at HTFL (2).
If the generalized HTFL does not account for the timeline of infection, then, assuming that t = ∞ and τ = 0, we will arrive at HFL (1):
The list of quantitative characteristics of pathogen virulence in the case of exposure to one pathogen, their probability and physical interpretation are provided in table.
Thus, the problem formulated at the beginning of this study is now completely theoretically solved.
Virulence depends on the species and strain of the studied pathogen, the route of entry (inhalation, ingestion, through mucous membranes) and the type of the biological object exposed to the pathogen (adults, children, the elderly, individuals with chronic conditions). Virulence should be experimentally assessed at the lab using model objects. The obtained results are then expected to be translated to humans.
Methods used to determine quantitative characteristics of virulence have been subjected to critical analysis. Among such methods are Kärber’s method [9], Finney’s probit analysis [10] and Bliss’s probit analysis [11]. Using the method of moments, maximal likelihood estimation and the method of least squares, researchers designed ways to measure 9 toxicological (virological) parameters of bioactive agents (pathogens) based on primary data from laboratory studies on model objects [8].
There is another important issue that needs to be discussed. The laws covered by this study are referred to as conditional static (deterministic) laws. In real life, the infective pathogen dose is a stochastic variable due to a number of subjective and objective reasons [8]. At the same time, quantitative characteristics of virulence are population parameters, i.e. deterministic variables. On the other hand, given the methods for their determination, they are estimates of the general population parameters and, therefore, are continuous random variables (this is a fundamental property of estimates).
Therefore, the studied probabilities of infection are functions of continuous random variables and are stochastic themselves. This raises the question of accounting for the random nature of variables in the laws described above. This problem can be discussed and solved under the stochastic theory of infection, the emergent, independent field of research [8, 12–17].
The literature offers a wealth of data on the incubation period: its minimum t min, maximum t max and sometimes average duration [18, 19]. To a first approximation, such data provide an insight into the temporal characteristics of virulence. Assuming that the minimum and maximum duration of the incubation period reported by the literature are in agreement with the 0.95 probability of random incubation period duration falling within this range, the following quantitative characteristics can be calculated:
However, more accurate estimates can be obtained in special experiments on model objects, followed by their translation to humans [8].
DISCUSSION
Our theoretical research allowed us to find the laws describing the probability of infection after exposure to one pathogen type, depending on the infective dose and considering the temporal characteristics of a given infection. The correctness of these laws was confirmed by dimensional analysis and their correct behavior in limiting and special cases.
An important practical implication of this theoretical research is the complete list of quantitative characteristics of virulence. It is important to know 9 or 5 quantitative characteristics of virulence, for reversible and irreversible effects, respectively.
Today, these quantitative characteristics are almost unknown, which is a serious setback for accurate epidemic modeling.
At present, the probability of establishing an infection is described based on the number of contacts between susceptibles and infectives [2, 3, 20, 21], which is wrong in principle.
CONCLUSION
We have constructed the hazard factor law, the hazard time-factor law and the generalized time-factor laws describing the probability of infection in humans and non-human biological objects (like agricultural animals) following exposure to one as opposed to a variety of pathogens. These laws help in solving practical tasks and should lie at the core of mathematical epidemiological modeling.
In order to successfully solve practical epidemiological tasks, further research should focus on identifying all quantitative characteristics of pathogens for every route of entry into the body and the obtained data should be compiled into a comprehensive database.